v1.1 release
This commit is contained in:
Родитель
1110f54277
Коммит
5f53847190
18
README.md
18
README.md
|
@ -42,9 +42,10 @@ For those interested in accessing the previous MegaDetector repository, which ut
|
|||
|
||||
### 🎉🎉🎉 Pytorch-Wildlife Version 1.1.0 is out!
|
||||
- MegaDetectorV6 is finally out! Please refer to our [next section](#racing_cardashdash-megadetectorv6-smaller-better-and-faster) and our [release page](https://github.com/microsoft/CameraTraps/releases) for more details!
|
||||
- We have incorporated a point-based overhead animal detection model into our model zoo called [HerdNet (Delplanque et al. 2022)](https://www.sciencedirect.com/science/article/pii/S092427162300031X?via%3Dihub). This is the first third-party model in Pytorch-Wildlife and the foundation of our expansion to overhead/aerial animal detection and classification. Please see our [HerdNet demo](demo/image_detection_demo_herdnet.ipynb) on how to use it!
|
||||
- We have incorporated a point-based overhead animal detection model into our model zoo called [HerdNet (Delplanque et al. 2022)](https://www.sciencedirect.com/science/article/pii/S092427162300031X?via%3Dihub). Two model weights are incorporated in this release, `HerdNet-general` (their default weights) and `HerdNet-ennedi` (their model trained on Ennedi 2019 datasets). More details can be found [here](PytorchWildlife/models/detection/herdnet/Herdnet.md) and in their original [repo](https://github.com/Alexandre-Delplanque/HerdNet). This is the first third-party model in Pytorch-Wildlife and the foundation of our expansion to overhead/aerial animal detection and classification. Please see our [HerdNet demo](demo/image_detection_demo_herdnet.ipynb) on how to use it!
|
||||
- You can now load custom weights you fine-tuned on your own datasets using the [finetuning module](PW_FT_classification) directly in the Pytorch-Wildlife pipeline! Please see the [demo](demo/custom_weight_loading_v6.ipynb) on how to do it. You can also load it in our Gradio app!
|
||||
- You can now automatically separate your image detections into folders based on detection results! Please see our [folder separation demo](demo/image_separation_demo_v6.ipynb) on how to do it. You can also test it in our Gradio demo!
|
||||
- We have also simplified the batch detection pipeline. Now we do not need to define pytorch datasets and dataloaders specifically. Please make sure to change your code and check our [release notes]() and our [new demo](demo/image_demo.py#58) for more details.
|
||||
|
||||
<details>
|
||||
<summary><font size="3">👉 Click for more updates</font></summary>
|
||||
|
@ -55,7 +56,7 @@ For those interested in accessing the previous MegaDetector repository, which ut
|
|||
|
||||
### :racing_car::dash::dash: MegaDetectorV6: SMALLER, BETTER, and FASTER!
|
||||
|
||||
After a few months of public beta testing, we are finally ready to officially release our 6th version of MegaDetector, MegaDetectorV6! In the next generation of MegaDetector, we are focusing on computational efficiency, performance, mordernizing of model architectures, and licensing. We have trained multiple new models using different model architectures, including Yolo-v9 and RT-Detr. We have a [rolling release schedule](#mag-model-zoo) for different versions of MegaDetectorV6, and in the first step, we are releasing the compact version of MegaDetectorV6 with Yolo-v9 (MDv6-ultralytics-yolov9-c).
|
||||
After a few months of public beta testing, we are finally ready to officially release our 6th version of MegaDetector, MegaDetectorV6! In the next generation of MegaDetector, we are focusing on computational efficiency, performance, mordernizing of model architectures, and licensing. We have trained multiple new models using different model architectures, including Yolo-v9, Yolo-v11, and RT-Detr for maximum user flexibility. We have a [rolling release schedule](#mag-model-zoo) for different versions of MegaDetectorV6, and in the first step, we are releasing the compact version of MegaDetectorV6 with Yolo-v9 (MDv6-ultralytics-yolov9-compact, MDv6-c in short). From now on, we encourage our users to use MegaDetectorV6 as their default animal detection model.
|
||||
|
||||
This MDv6-c model is optimized for performance and low-budget devices. It has only ***one-sixth (SMALLER)*** of the parameters of the previous MegaDetectorV5 and exhibits ***12% higher recall (BETTER)*** on animal detection in our validation datasets. In other words, MDv6-c has significantly fewer false negatives when detecting animals, making it a more robust animal detection model than MegaDetectorV5. Furthermore, one of our testers reported that the speed of MDv6-c is at least ***5 times FASTER*** than MegaDetectorV5 on their datasets.
|
||||
|
||||
|
@ -76,15 +77,18 @@ We want to make Pytorch-Wildlife a platform where different models with differen
|
|||
|
||||
In addition, since the **Pytorch-Wildlife** package is under MIT, all the utility functions, including data pre-/post-processing functions and model fine-tuning functions in this packages are under MIT as well.
|
||||
|
||||
### :mag: Model Zoo
|
||||
### :mag: Model Zoo and Release Schedules
|
||||
|
||||
#### Detection models
|
||||
|Models|Licence|Release|
|
||||
|---|---|---|
|
||||
|MegaDetectorV5|AGPL-3.0|Released|
|
||||
|MegaDetectroV6-Ultralytics-YoloV9-Compact|AGPL-3.0|Released|
|
||||
|HerdNet|CC BY-NC-SA-4.0|Released|
|
||||
|HerdNet-general|CC BY-NC-SA-4.0|Released|
|
||||
|HerdNet-ennedi|CC BY-NC-SA-4.0|Released|
|
||||
|MegaDetectroV6-Ultralytics-YoloV9-Extra|AGPL-3.0|November 2024|
|
||||
|MegaDetectroV6-Ultralytics-YoloV11-Compact (even smaller and no NMS)|AGPL-3.0|November 2024|
|
||||
|MegaDetectroV6-Ultralytics-YoloV11-Extra (even smaller and no NMS)|AGPL-3.0|November 2024|
|
||||
|MegaDetectroV6-MIT-YoloV9-Compact|MIT|November 2024|
|
||||
|MegaDetectroV6-MIT-YoloV9-Extra|MIT|November 2024|
|
||||
|MegaDetectroV6-Apache-RTDetr-Compact|Apache|December 2024|
|
||||
|
@ -176,15 +180,15 @@ Let's shape the future of wildlife research, together! 🙌
|
|||
|
||||
## 🖼️ Examples
|
||||
|
||||
### Image detection using `MegaDetector v5`
|
||||
### Image detection using `MegaDetector`
|
||||
<img src="https://microsoft.github.io/CameraTraps/assets/animal_det_1.JPG" alt="animal_det_1" width="400"/><br>
|
||||
*Credits to Universidad de los Andes, Colombia.*
|
||||
|
||||
### Image classification with `MegaDetector v5` and `AI4GAmazonRainforest`
|
||||
### Image classification with `MegaDetector` and `AI4GAmazonRainforest`
|
||||
<img src="https://microsoft.github.io/CameraTraps/assets/animal_clas_1.png" alt="animal_clas_1" width="500"/><br>
|
||||
*Credits to Universidad de los Andes, Colombia.*
|
||||
|
||||
### Opossum ID with `MegaDetector v5` and `AI4GOpossum`
|
||||
### Opossum ID with `MegaDetector` and `AI4GOpossum`
|
||||
<img src="https://microsoft.github.io/CameraTraps/assets/opossum_det.png" alt="opossum_det" width="500"/><br>
|
||||
*Credits to the Agency for Regulation and Control of Biosecurity and Quarantine for Galápagos (ABG), Ecuador.*
|
||||
|
||||
|
|
Двоичные данные
assets/gradio_UI.png
Двоичные данные
assets/gradio_UI.png
Двоичный файл не отображается.
До Ширина: | Высота: | Размер: 589 KiB После Ширина: | Высота: | Размер: 983 KiB |
187
megadetector.md
187
megadetector.md
|
@ -16,51 +16,6 @@
|
|||
<br><br>
|
||||
</div>
|
||||
|
||||
|
||||
## 📣 Announcement
|
||||
|
||||
### 🤜🤛 Collaboration with EcoAssist!
|
||||
We are thrilled to announce our collaboration with [EcoAssist](https://addaxdatascience.com/ecoassist/#spp-models)---a powerful user interface software that enables users to directly load models from the PyTorch-Wildlife model zoo for image analysis on local computers. With EcoAssist, you can now utilize MegaDetectorV5 and the classification models---AI4GAmazonRainforest and AI4GOpossum---for automatic animal detection and identification, alongside a comprehensive suite of pre- and post-processing tools. This partnership aims to enhance the overall user experience with PyTorch-Wildlife models for a general audience. We will work closely to bring more features together for more efficient and effective wildlife analysis in the future.
|
||||
|
||||
### 🏎️💨💨 SMALLER, BETTER, and FASTER! MegaDetectorV6 public beta testing started!
|
||||
The public beta testing for MegaDetectorV6 has officially started! In the next generation of MegaDetector, we are focusing on computational efficiency and performance. We have trained multiple new models using the latest YOLO-v9 architecture, and in the public beta testing, we will allow people to test the compact version of MegaDetectorV6 (MDv6-c). We want to make sure these models work as expected on real-world datasets.
|
||||
|
||||
This MDv6-c model has only ***one-sixth (SMALLER)*** of the parameters of the current MegaDetectorV5 and exhibits ***12% higher recall (BETTER)*** on animal detection in our validation datasets. In other words, MDv6-c has significantly fewer false negatives when detecting animals, making it a more robust animal detection model than MegaDetectorV5. Furthermore, one of our testers reported that the speed of MDv6-c is at least ***5 times FASTER*** than MegaDetectorV5 on their datasets.
|
||||
|
||||
|Models|Parameters|Precision|Recall|
|
||||
|---|---|---|---|
|
||||
|MegaDetectorV5|121M|0.96|0.73|
|
||||
|MegaDetectroV6-c|22M|0.92|0.85|
|
||||
|
||||
We are also working on an extra-large version of MegaDetectorV6 for optimal performance and a transformer-based model using the RT-Detr architecture to prepare ourselves for the future of transformers. These models will be available in the official release of MegaDetectorV6.
|
||||
|
||||
>If you want to join the beta testing, please come to our discord channel and DM the admins there: [](https://discord.gg/TeEVxzaYtm)
|
||||
|
||||
### 🎉 Pytorch-Wildlife ready for citation
|
||||
In addition, we have recently published a [summary paper on Pytorch-Wildlife](https://arxiv.org/abs/2405.12930). The paper has been accepted as an oral presentation at the [CV4Animals workshop](https://www.cv4animals.com/) at this year's CVPR. Please feel free to [cite us!](#cite-us)
|
||||
|
||||
|
||||
## ✅ Feature highlights (Version 1.0.2.15)
|
||||
- [x] Added a file separation function. You can now automatically separate your files between animals and non-animals into different folders using our `detection_folder_separation` function. Please see the [Python demo file](demo/image_separation_demo.py) and [Jupyter demo](demo/image_separation_demo.ipynb)!
|
||||
- [x] 🥳 Added Timelapse compatibility! Check the [Gradio interface](INSTALLATION.md) or [notebooks](https://github.com/microsoft/CameraTraps/blob/main/demo/image_detection_demo.ipynb).
|
||||
<details>
|
||||
<summary><font size="3">👉 Click for more</font></summary>
|
||||
<li> CUDA 12.x compatibility. <br>
|
||||
<li> Added Google Colab demos. <br>
|
||||
<li> Added Snapshot Serengeti classification model into the model zoo. <br>
|
||||
<li> Added Classification fine-tuning module. <br>
|
||||
<li> Added a Docker Image for ease of installation. <br>
|
||||
</details>
|
||||
|
||||
## 🔥 Future highlights
|
||||
- [ ] MegaDetectorV6 with multiple model sizes for both optimized performance and low-budget devices like camera systems (***Public beta testing has started!!***).
|
||||
- [ ] Supervision 0.19+ and Python 3.10+ compatibility.
|
||||
- [ ] A detection model fine-tuning module to fine-tune your own detection model for Pytorch-Wildlife.
|
||||
- [ ] Direct LILA connection for more training/validation data.
|
||||
- [ ] More pretrained detection and classification models to expand the current model zoo.
|
||||
|
||||
To check the full version of the roadmap with completed tasks and long term goals, please click [here!](roadmaps.md).
|
||||
|
||||
## 🐾 Introduction
|
||||
|
||||
At the core of our mission is the desire to create a harmonious space where conservation scientists from all over the globe can unite. Where they're able to share, grow, use datasets and deep learning architectures for wildlife conservation.
|
||||
|
@ -74,20 +29,85 @@ pip install PytorchWildlife
|
|||
To use the newest version of MegaDetector with all the existing functionalities, you can use our [Hugging Face interface](https://huggingface.co/spaces/ai-for-good-lab/pytorch-wildlife) or simply load the model with **Pytorch-Wildlife**. The weights will be automatically downloaded:
|
||||
```python
|
||||
from PytorchWildlife.models import detection as pw_detection
|
||||
detection_model = pw_detection.MegaDetectorV5()
|
||||
detection_model = pw_detection.MegaDetectorV6()
|
||||
```
|
||||
|
||||
For those interested in accessing the previous MegaDetector repository, which utilizes the same `MegaDetector v5` model weights and was primarily developed by Dan Morris during his time at Microsoft, please visit the [archive](https://github.com/microsoft/CameraTraps/blob/main/archive) directory, or you can visit this [forked repository](https://github.com/agentmorris/MegaDetector/tree/main) that Dan Morris is actively maintaining.
|
||||
For those interested in accessing the previous MegaDetector repository, which utilizes the same `MegaDetectorV5` model weights and was primarily developed by Dan Morris during his time at Microsoft, please visit the [archive](https://github.com/microsoft/CameraTraps/blob/main/archive) directory, or you can visit this [forked repository](https://github.com/agentmorris/MegaDetector/tree/main) that Dan Morris is actively maintaining.
|
||||
|
||||
>[!TIP]
|
||||
>If you have any questions regarding MegaDetector and Pytorch-Wildlife, please [email us](zhongqimiao@microsoft.com) or join us in our discord channel: [](https://discord.gg/TeEVxzaYtm)
|
||||
|
||||
## 👋 Welcome to Pytorch-Wildlife Version 1.0
|
||||
|
||||
## 📣 Announcements
|
||||
|
||||
### 🎉🎉🎉 Pytorch-Wildlife Version 1.1.0 is out!
|
||||
- MegaDetectorV6 is finally out! Please refer to our [next section](#racing_cardashdash-megadetectorv6-smaller-better-and-faster) and our [release page](https://github.com/microsoft/CameraTraps/releases) for more details!
|
||||
- We have incorporated a point-based overhead animal detection model into our model zoo called [HerdNet (Delplanque et al. 2022)](https://www.sciencedirect.com/science/article/pii/S092427162300031X?via%3Dihub). Two model weights are incorporated in this release, `HerdNet-general` (their default weights) and `HerdNet-ennedi` (their model trained on Ennedi 2019 datasets). More details can be found [here](PytorchWildlife/models/detection/herdnet/Herdnet.md) and in their original [repo](https://github.com/Alexandre-Delplanque/HerdNet). This is the first third-party model in Pytorch-Wildlife and the foundation of our expansion to overhead/aerial animal detection and classification. Please see our [HerdNet demo](demo/image_detection_demo_herdnet.ipynb) on how to use it!
|
||||
- You can now load custom weights you fine-tuned on your own datasets using the [finetuning module](PW_FT_classification) directly in the Pytorch-Wildlife pipeline! Please see the [demo](demo/custom_weight_loading_v6.ipynb) on how to do it. You can also load it in our Gradio app!
|
||||
- You can now automatically separate your image detections into folders based on detection results! Please see our [folder separation demo](demo/image_separation_demo_v6.ipynb) on how to do it. You can also test it in our Gradio demo!
|
||||
- We have also simplified the batch detection pipeline. Now we do not need to define pytorch datasets and dataloaders specifically. Please make sure to change your code and check our [release notes]() and our [new demo](demo/image_demo.py#58) for more details.
|
||||
|
||||
<details>
|
||||
<summary><font size="3">👉 Click for more updates</font></summary>
|
||||
<li> Issues [#523](https://github.com/microsoft/CameraTraps/issues/523), [#524](https://github.com/microsoft/CameraTraps/issues/524) and [#526](https://github.com/microsoft/CameraTraps/issues/526) have been solved!
|
||||
<li> PyTorchWildlife is now compatible with Supervision 0.23+ and Python 3.10+!
|
||||
<li> CUDA 12.x compatibility. <br>
|
||||
</details>
|
||||
|
||||
### :racing_car::dash::dash: MegaDetectorV6: SMALLER, BETTER, and FASTER!
|
||||
|
||||
After a few months of public beta testing, we are finally ready to officially release our 6th version of MegaDetector, MegaDetectorV6! In the next generation of MegaDetector, we are focusing on computational efficiency, performance, mordernizing of model architectures, and licensing. We have trained multiple new models using different model architectures, including Yolo-v9, Yolo-v11, and RT-Detr for maximum user flexibility. We have a [rolling release schedule](#mag-model-zoo) for different versions of MegaDetectorV6, and in the first step, we are releasing the compact version of MegaDetectorV6 with Yolo-v9 (MDv6-ultralytics-yolov9-compact, MDv6-c in short). From now on, we encourage our users to use MegaDetectorV6 as their default animal detection model.
|
||||
|
||||
This MDv6-c model is optimized for performance and low-budget devices. It has only ***one-sixth (SMALLER)*** of the parameters of the previous MegaDetectorV5 and exhibits ***12% higher recall (BETTER)*** on animal detection in our validation datasets. In other words, MDv6-c has significantly fewer false negatives when detecting animals, making it a more robust animal detection model than MegaDetectorV5. Furthermore, one of our testers reported that the speed of MDv6-c is at least ***5 times FASTER*** than MegaDetectorV5 on their datasets.
|
||||
|
||||
|Models|Parameters|Precision|Recall|
|
||||
|---|---|---|---|
|
||||
|MegaDetectorV5|121M|0.96|0.73|
|
||||
|MegaDetectroV6-c|22M|0.92|0.85|
|
||||
|
||||
Learn how to use MegaDetectorV6 in our [image demo](demo/image_detection_demo_v6.ipynb) and [video demo](demo/video_detection_demo_v6.ipynb).
|
||||
|
||||
### :bangbang: Model licensing `(IMPORTANT!!)`
|
||||
|
||||
The **Pytorch-Wildlife** package is under MIT, however some of the models in the model zoo are not. For example, MegaDetectorV5, which is trained using the Ultralytics package, is under AGPL-3.0, and is not for closed-source comercial uses.
|
||||
> [!IMPORTANT]
|
||||
> THIS IS TRUE TO ALL EXISTING MEGADETECTORV5 MODELS IN ALL EXISTING FORKS THAT ARE TRAINED USING YOLOV5, AN ULTRALYTICS-DEVELOPED MODEL.
|
||||
|
||||
We want to make Pytorch-Wildlife a platform where different models with different licenses can be hosted and want to enable different usecases. To reduce user confusions, in our [model zoo](#mag-model-zoo) section, we list all existing and planed future models in our model zoo, their corresponding license, and release schedules.
|
||||
|
||||
In addition, since the **Pytorch-Wildlife** package is under MIT, all the utility functions, including data pre-/post-processing functions and model fine-tuning functions in this packages are under MIT as well.
|
||||
|
||||
### :mag: Model Zoo and Release Schedules
|
||||
|
||||
#### Detection models
|
||||
|Models|Licence|Release|
|
||||
|---|---|---|
|
||||
|MegaDetectorV5|AGPL-3.0|Released|
|
||||
|MegaDetectroV6-Ultralytics-YoloV9-Compact|AGPL-3.0|Released|
|
||||
|HerdNet-general|CC BY-NC-SA-4.0|Released|
|
||||
|HerdNet-ennedi|CC BY-NC-SA-4.0|Released|
|
||||
|MegaDetectroV6-Ultralytics-YoloV9-Extra|AGPL-3.0|November 2024|
|
||||
|MegaDetectroV6-Ultralytics-YoloV11-Compact (even smaller and no NMS)|AGPL-3.0|November 2024|
|
||||
|MegaDetectroV6-Ultralytics-YoloV11-Extra (even smaller and no NMS)|AGPL-3.0|November 2024|
|
||||
|MegaDetectroV6-MIT-YoloV9-Compact|MIT|November 2024|
|
||||
|MegaDetectroV6-MIT-YoloV9-Extra|MIT|November 2024|
|
||||
|MegaDetectroV6-Apache-RTDetr-Compact|Apache|December 2024|
|
||||
|MegaDetectroV6-Apache-RTDetr-Extra|Apache|December 2024|
|
||||
|
||||
#### Classification models
|
||||
|Models|Licence|Release|
|
||||
|---|---|---|
|
||||
|AI4G-Oppossum|MIT|Released|
|
||||
|AI4G-Amazon|MIT|Released|
|
||||
|AI4G-Serengeti|MIT|Released|
|
||||
|
||||
|
||||
## 👋 Welcome to Pytorch-Wildlife
|
||||
|
||||
**PyTorch-Wildlife** is a platform to create, modify, and share powerful AI conservation models. These models can be used for a variety of applications, including camera trap images, overhead images, underwater images, or bioacoustics. Your engagement with our work is greatly appreciated, and we eagerly await any feedback you may have.
|
||||
|
||||
|
||||
The **Pytorch-Wildlife** library allows users to directly load the `MegaDetector v5` model weights for animal detection. We've fully refactored our codebase, prioritizing ease of use in model deployment and expansion. In addition to `MegaDetector v5`, **Pytorch-Wildlife** also accommodates a range of classification weights, such as those derived from the Amazon Rainforest dataset and the Opossum classification dataset. Explore the codebase and functionalities of **Pytorch-Wildlife** through our interactive [HuggingFace web app](https://huggingface.co/spaces/AndresHdzC/pytorch-wildlife) or local [demos and notebooks](https://github.com/microsoft/CameraTraps/tree/main/demo), designed to showcase the practical applications of our enhancements at [PyTorchWildlife](https://github.com/microsoft/CameraTraps/blob/main/INSTALLATION.md). You can find more information in our [documentation](https://cameratraps.readthedocs.io/en/latest/).
|
||||
The **Pytorch-Wildlife** library allows users to directly load the `MegaDetector` model weights for animal detection. We've fully refactored our codebase, prioritizing ease of use in model deployment and expansion. In addition to `MegaDetector`, **Pytorch-Wildlife** also accommodates a range of classification weights, such as those derived from the Amazon Rainforest dataset and the Opossum classification dataset. Explore the codebase and functionalities of **Pytorch-Wildlife** through our interactive [HuggingFace web app](https://huggingface.co/spaces/AndresHdzC/pytorch-wildlife) or local [demos and notebooks](https://github.com/microsoft/CameraTraps/tree/main/demo), designed to showcase the practical applications of our enhancements at [PyTorchWildlife](https://github.com/microsoft/CameraTraps/blob/main/INSTALLATION.md). You can find more information in our [documentation](https://cameratraps.readthedocs.io/en/latest/).
|
||||
|
||||
👇 Here is a brief example on how to perform detection and classification on a single image using `PyTorch-wildlife`
|
||||
```python
|
||||
|
@ -98,7 +118,7 @@ from PytorchWildlife.models import classification as pw_classification
|
|||
img = np.random.randn(3, 1280, 1280)
|
||||
|
||||
# Detection
|
||||
detection_model = pw_detection.MegaDetectorV5() # Model weights are automatically downloaded.
|
||||
detection_model = pw_detection.MegaDetectorV6() # Model weights are automatically downloaded.
|
||||
detection_result = detection_model.single_image_detection(img)
|
||||
|
||||
#Classification
|
||||
|
@ -114,7 +134,7 @@ Please refer to our [installation guide](https://github.com/microsoft/CameraTrap
|
|||
|
||||
## 🕵️ Explore Pytorch-Wildlife and MegaDetector with our Demo User Interface
|
||||
|
||||
If you want to directly try **Pytorch-Wildlife** with the AI models available, including `MegaDetector v5`, you can use our [**Gradio** interface](https://github.com/microsoft/CameraTraps/tree/main/demo). This interface allows users to directly load the `MegaDetector v5` model weights for animal detection. In addition, **Pytorch-Wildlife** also has two classification models in our initial version. One is trained from an Amazon Rainforest camera trap dataset and the other from a Galapagos opossum classification dataset (more details of these datasets will be published soon). To start, please follow the [installation instructions](https://github.com/microsoft/CameraTraps/blob/main/INSTALLATION.md) on how to run the Gradio interface! We also provide multiple [**Jupyter** notebooks](https://github.com/microsoft/CameraTraps/tree/main/demo) for demonstration.
|
||||
If you want to directly try **Pytorch-Wildlife** with the AI models available, including `MegaDetector`, you can use our [**Gradio** interface](https://github.com/microsoft/CameraTraps/tree/main/demo). This interface allows users to directly load the `MegaDetector` model weights for animal detection. In addition, **Pytorch-Wildlife** also has two classification models in our initial version. One is trained from an Amazon Rainforest camera trap dataset and the other from a Galapagos opossum classification dataset (more details of these datasets will be published soon). To start, please follow the [installation instructions](https://github.com/microsoft/CameraTraps/blob/main/INSTALLATION.md) on how to run the Gradio interface! We also provide multiple [**Jupyter** notebooks](https://github.com/microsoft/CameraTraps/tree/main/demo) for demonstration.
|
||||
|
||||
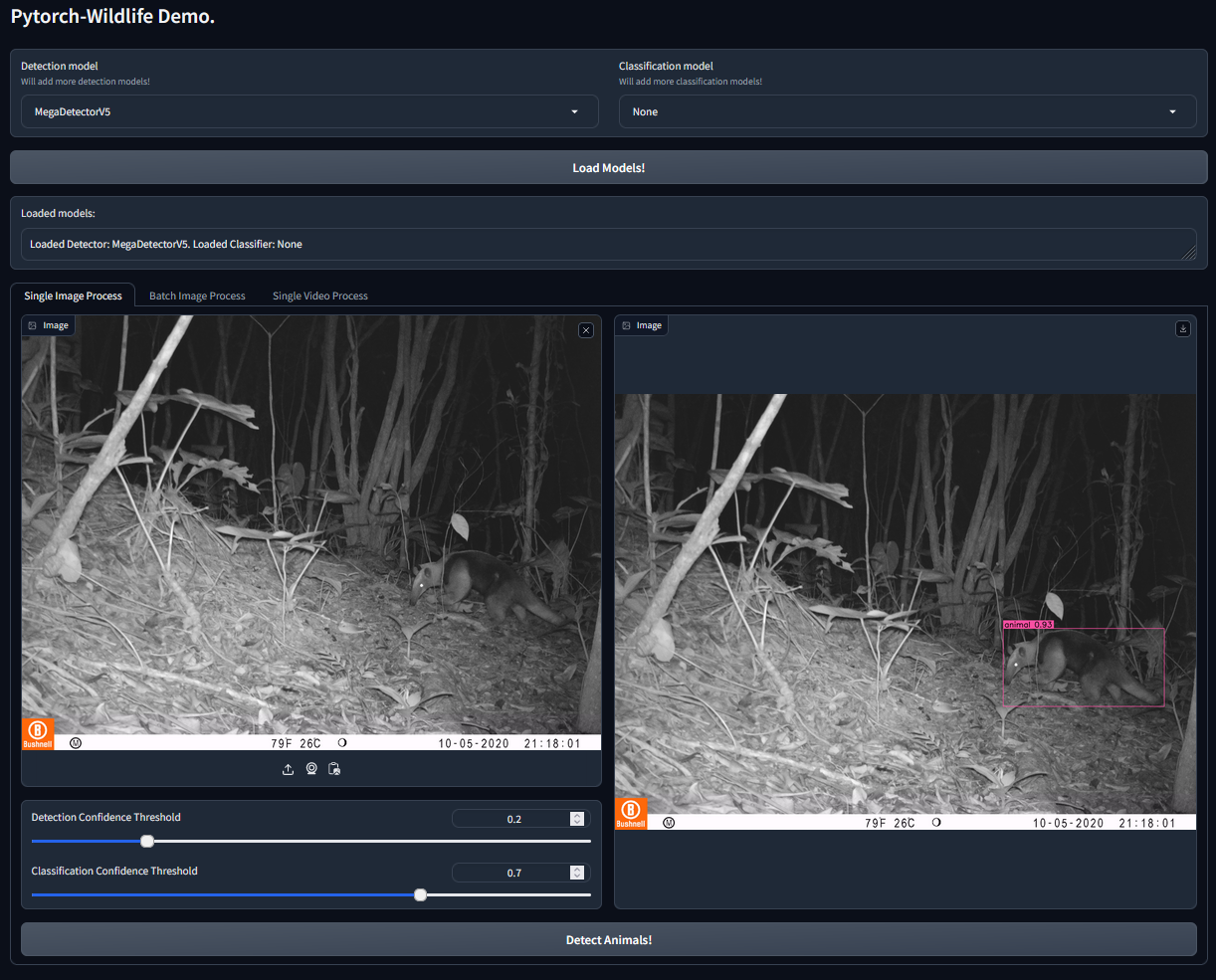
|
||||
|
||||
|
@ -137,7 +157,7 @@ If you want to directly try **Pytorch-Wildlife** with the AI models available, i
|
|||
|
||||
|
||||
### 🚀 Inaugural Model:
|
||||
We're kickstarting with YOLO as our first available model, complemented by pre-trained weights from `MegaDetector v5`. This is the same `MegaDetector v5` model from the previous repository.
|
||||
We're kickstarting with YOLO as our first available model, complemented by pre-trained weights from `MegaDetector`. We have `MegaDetectorV5`, which is the same `MegaDetector v5` model from the previous repository, and many different versions of `MegaDetectorV6` for different usecases.
|
||||
|
||||
|
||||
### 📚 Expandable Repository:
|
||||
|
@ -158,61 +178,34 @@ If you want to directly try **Pytorch-Wildlife** with the AI models available, i
|
|||
|
||||
Let's shape the future of wildlife research, together! 🙌
|
||||
|
||||
|
||||
### 📈 Progress on core tasks
|
||||
|
||||
<details>
|
||||
<summary> <font size="3"> ▪️ Packaging </font> </summary>
|
||||
|
||||
- [ ] Animal detection fine-tuning<br>
|
||||
- [x] MegaDetectorV5 integration<br>
|
||||
- [ ] MegaDetectorV6 integration<br>
|
||||
- [x] User submitted weights<br>
|
||||
- [x] Animal classification fine-tuning<br>
|
||||
- [x] Amazon Rainforest classification<br>
|
||||
- [x] Amazon Opossum classification<br>
|
||||
- [ ] User submitted weights<br>
|
||||
</details><br>
|
||||
|
||||
<details>
|
||||
<summary><font size="3">▪️ Utility Toolkit</font></summary>
|
||||
|
||||
- [x] Visualization tools<br>
|
||||
- [x] MegaDetector utils<br>
|
||||
- [ ] User submitted utils<br>
|
||||
</details><br>
|
||||
|
||||
<details>
|
||||
<summary><font size="3">▪️ Datasets</font></summary>
|
||||
|
||||
- [ ] Animal Datasets<br>
|
||||
- [ ] LILA datasets<br>
|
||||
</details><br>
|
||||
|
||||
<details>
|
||||
<summary><font size="3">▪️ Accessibility</font></summary>
|
||||
|
||||
- [x] Basic user interface for demonstration<br>
|
||||
- [ ] UI Dev tools<br>
|
||||
- [ ] List of available UIs<br>
|
||||
</details><br>
|
||||
|
||||
|
||||
## 🖼️ Examples
|
||||
|
||||
### Image detection using `MegaDetector v5`
|
||||
### Image detection using `MegaDetector`
|
||||
<img src="https://microsoft.github.io/CameraTraps/assets/animal_det_1.JPG" alt="animal_det_1" width="400"/><br>
|
||||
*Credits to Universidad de los Andes, Colombia.*
|
||||
|
||||
### Image classification with `MegaDetector v5` and `AI4GAmazonRainforest`
|
||||
### Image classification with `MegaDetector` and `AI4GAmazonRainforest`
|
||||
<img src="https://microsoft.github.io/CameraTraps/assets/animal_clas_1.png" alt="animal_clas_1" width="500"/><br>
|
||||
*Credits to Universidad de los Andes, Colombia.*
|
||||
|
||||
### Opossum ID with `MegaDetector v5` and `AI4GOpossum`
|
||||
### Opossum ID with `MegaDetector` and `AI4GOpossum`
|
||||
<img src="https://microsoft.github.io/CameraTraps/assets/opossum_det.png" alt="opossum_det" width="500"/><br>
|
||||
*Credits to the Agency for Regulation and Control of Biosecurity and Quarantine for Galápagos (ABG), Ecuador.*
|
||||
|
||||
## Cite us
|
||||
## 🔥 Future highlights
|
||||
- [ ] A detection model fine-tuning module to fine-tune your own detection model for Pytorch-Wildlife.
|
||||
- [ ] Direct LILA connection for more training/validation data.
|
||||
- [ ] More pretrained detection and classification models to expand the current model zoo.
|
||||
|
||||
To check the full version of the roadmap with completed tasks and long term goals, please click [here!](roadmaps.md).
|
||||
|
||||
## 🤜🤛 Collaboration with EcoAssist!
|
||||
We are thrilled to announce our collaboration with [EcoAssist](https://addaxdatascience.com/ecoassist/#spp-models)---a powerful user interface software that enables users to directly load models from the PyTorch-Wildlife model zoo for image analysis on local computers. With EcoAssist, you can now utilize MegaDetectorV5 and the classification models---AI4GAmazonRainforest and AI4GOpossum---for automatic animal detection and identification, alongside a comprehensive suite of pre- and post-processing tools. This partnership aims to enhance the overall user experience with PyTorch-Wildlife models for a general audience. We will work closely to bring more features together for more efficient and effective wildlife analysis in the future.
|
||||
|
||||
|
||||
## :fountain_pen: Cite us!
|
||||
We have recently published a [summary paper on Pytorch-Wildlife](https://arxiv.org/abs/2405.12930). The paper has been accepted as an oral presentation at the [CV4Animals workshop](https://www.cv4animals.com/) at this CVPR 2024. Please feel free to cite us!
|
||||
|
||||
```
|
||||
@misc{hernandez2024pytorchwildlife,
|
||||
title={Pytorch-Wildlife: A Collaborative Deep Learning Framework for Conservation},
|
||||
|
@ -374,5 +367,3 @@ Protected Areas Unit, Canadian Wildlife Service
|
|||
>[!IMPORTANT]
|
||||
>If you would like to be added to this list or have any questions regarding MegaDetector and Pytorch-Wildlife, please [email us](zhongqimiao@microsoft.com) or join us in our Discord channel: [](https://discord.gg/TeEVxzaYtm)
|
||||
|
||||
|
||||
|
||||
|
|
15
setup.py
15
setup.py
|
@ -4,7 +4,7 @@ with open('README.md', encoding="utf8") as file:
|
|||
long_description = file.read()
|
||||
setup(
|
||||
name='PytorchWildlife',
|
||||
version='1.0.2.17',
|
||||
version='1.1.0',
|
||||
packages=find_packages(),
|
||||
url='https://github.com/microsoft/CameraTraps/',
|
||||
license='MIT',
|
||||
|
@ -14,14 +14,13 @@ setup(
|
|||
long_description=long_description,
|
||||
long_description_content_type='text/markdown',
|
||||
install_requires=[
|
||||
'numpy',
|
||||
'torch==1.10.1',
|
||||
'torchvision==0.11.2',
|
||||
'torchaudio==0.10.1',
|
||||
'tqdm==4.66.1',
|
||||
'Pillow==10.1.0',
|
||||
'torch',
|
||||
'torchvision',
|
||||
'torchaudio',
|
||||
'tqdm',
|
||||
'Pillow',
|
||||
'supervision==0.23.0',
|
||||
'gradio==4.8.0',
|
||||
'gradio',
|
||||
'ultralytics-yolov5',
|
||||
'ultralytics',
|
||||
'chardet',
|
||||
|
|
Загрузка…
Ссылка в новой задаче