371 строка
14 KiB
Plaintext
371 строка
14 KiB
Plaintext
{
|
|
"cells": [
|
|
{
|
|
"cell_type": "markdown",
|
|
"metadata": {
|
|
"pycharm": {
|
|
"is_executing": false,
|
|
"name": "#%% md\n"
|
|
}
|
|
},
|
|
"source": [
|
|
"## Tokenization doesn't have to be slow !\n",
|
|
"\n",
|
|
"### Introduction\n",
|
|
"\n",
|
|
"Before going deep into any Machine Learning or Deep Learning Natural Language Processing models, every practitioner\n",
|
|
"should find a way to map raw input strings to a representation understandable by a trainable model.\n",
|
|
"\n",
|
|
"One very simple approach would be to split inputs over every space and assign an identifier to each word. This approach\n",
|
|
"would look similar to the code below in python\n",
|
|
"\n",
|
|
"```python\n",
|
|
"s = \"very long corpus...\"\n",
|
|
"words = s.split(\" \") # Split over space\n",
|
|
"vocabulary = dict(enumerate(set(words))) # Map storing the word to it's corresponding id\n",
|
|
"```\n",
|
|
"\n",
|
|
"This approach might work well if your vocabulary remains small as it would store every word (or **token**) present in your original\n",
|
|
"input. Moreover, word variations like \"cat\" and \"cats\" would not share the same identifiers even if their meaning is \n",
|
|
"quite close.\n",
|
|
"\n",
|
|
"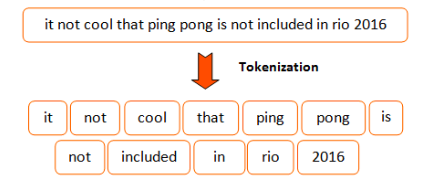\n",
|
|
"\n",
|
|
"### Subtoken Tokenization\n",
|
|
"\n",
|
|
"To overcome the issues described above, recent works have been done on tokenization, leveraging \"subtoken\" tokenization.\n",
|
|
"**Subtokens** extends the previous splitting strategy to furthermore explode a word into grammatically logicial sub-components learned\n",
|
|
"from the data.\n",
|
|
"\n",
|
|
"Taking our previous example of the words __cat__ and __cats__, a sub-tokenization of the word __cats__ would be [cat, ##s]. Where the prefix _\"##\"_ indicates a subtoken of the initial input. \n",
|
|
"Such training algorithms might extract sub-tokens such as _\"##ing\"_, _\"##ed\"_ over English corpus.\n",
|
|
"\n",
|
|
"As you might think of, this kind of sub-tokens construction leveraging compositions of _\"pieces\"_ overall reduces the size\n",
|
|
"of the vocabulary you have to carry to train a Machine Learning model. On the other side, as one token might be exploded\n",
|
|
"into multiple subtokens, the input of your model might increase and become an issue on model with non-linear complexity over the input sequence's length. \n",
|
|
" \n",
|
|
"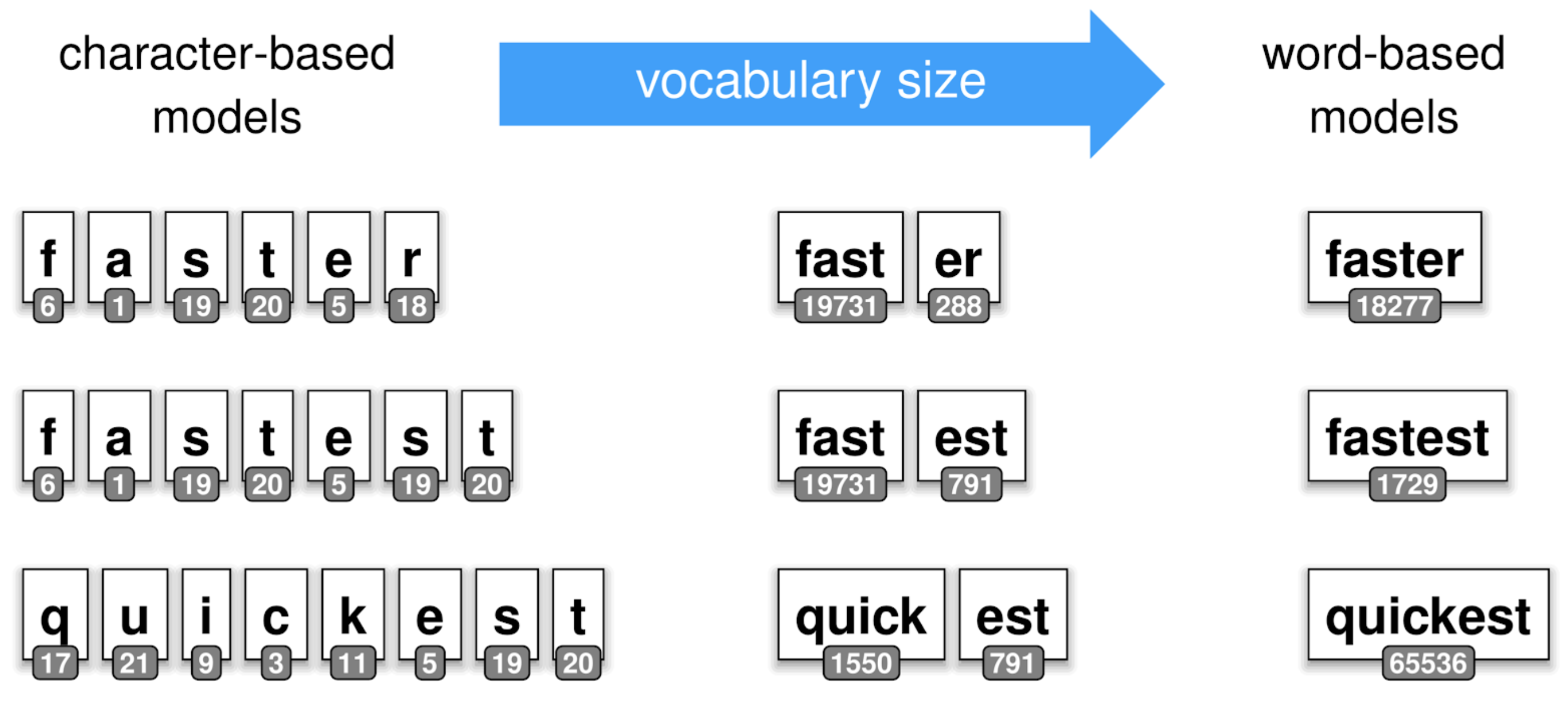\n",
|
|
" \n",
|
|
"Among all the tokenization algorithms, we can highlight a few subtokens algorithms used in Transformers-based SoTA models : \n",
|
|
"\n",
|
|
"- [Byte Pair Encoding (BPE) - Neural Machine Translation of Rare Words with Subword Units (Sennrich et al., 2015)](https://arxiv.org/abs/1508.07909)\n",
|
|
"- [Word Piece - Japanese and Korean voice search (Schuster, M., and Nakajima, K., 2015)](https://research.google/pubs/pub37842/)\n",
|
|
"- [Unigram Language Model - Subword Regularization: Improving Neural Network Translation Models with Multiple Subword Candidates (Kudo, T., 2018)](https://arxiv.org/abs/1804.10959)\n",
|
|
"- [Sentence Piece - A simple and language independent subword tokenizer and detokenizer for Neural Text Processing (Taku Kudo and John Richardson, 2018)](https://arxiv.org/abs/1808.06226)\n",
|
|
"\n",
|
|
"Going through all of them is out of the scope of this notebook, so we will just highlight how you can use them.\n",
|
|
"\n",
|
|
"### @huggingface/tokenizers library \n",
|
|
"Along with the transformers library, we @huggingface provide a blazing fast tokenization library\n",
|
|
"able to train, tokenize and decode dozens of Gb/s of text on a common multi-core machine.\n",
|
|
"\n",
|
|
"The library is written in Rust allowing us to take full advantage of multi-core parallel computations in a native and memory-aware way, on-top of which \n",
|
|
"we provide bindings for Python and NodeJS (more bindings may be added in the future). \n",
|
|
"\n",
|
|
"We designed the library so that it provides all the required blocks to create end-to-end tokenizers in an interchangeable way. In that sense, we provide\n",
|
|
"these various components: \n",
|
|
"\n",
|
|
"- **Normalizer**: Executes all the initial transformations over the initial input string. For example when you need to\n",
|
|
"lowercase some text, maybe strip it, or even apply one of the common unicode normalization process, you will add a Normalizer. \n",
|
|
"- **PreTokenizer**: In charge of splitting the initial input string. That's the component that decides where and how to\n",
|
|
"pre-segment the origin string. The simplest example would be like we saw before, to simply split on spaces.\n",
|
|
"- **Model**: Handles all the sub-token discovery and generation, this part is trainable and really dependant\n",
|
|
" of your input data.\n",
|
|
"- **Post-Processor**: Provides advanced construction features to be compatible with some of the Transformers-based SoTA\n",
|
|
"models. For instance, for BERT it would wrap the tokenized sentence around [CLS] and [SEP] tokens.\n",
|
|
"- **Decoder**: In charge of mapping back a tokenized input to the original string. The decoder is usually chosen according\n",
|
|
"to the `PreTokenizer` we used previously.\n",
|
|
"- **Trainer**: Provides training capabilities to each model.\n",
|
|
"\n",
|
|
"For each of the components above we provide multiple implementations:\n",
|
|
"\n",
|
|
"- **Normalizer**: Lowercase, Unicode (NFD, NFKD, NFC, NFKC), Bert, Strip, ...\n",
|
|
"- **PreTokenizer**: ByteLevel, WhitespaceSplit, CharDelimiterSplit, Metaspace, ...\n",
|
|
"- **Model**: WordLevel, BPE, WordPiece\n",
|
|
"- **Post-Processor**: BertProcessor, ...\n",
|
|
"- **Decoder**: WordLevel, BPE, WordPiece, ...\n",
|
|
"\n",
|
|
"All of these building blocks can be combined to create working tokenization pipelines. \n",
|
|
"In the next section we will go over our first pipeline."
|
|
]
|
|
},
|
|
{
|
|
"cell_type": "markdown",
|
|
"metadata": {
|
|
"pycharm": {
|
|
"name": "#%% md\n"
|
|
}
|
|
},
|
|
"source": [
|
|
"Alright, now we are ready to implement our first tokenization pipeline through `tokenizers`. \n",
|
|
"\n",
|
|
"For this, we will train a Byte-Pair Encoding (BPE) tokenizer on a quite small input for the purpose of this notebook.\n",
|
|
"We will work with [the file from Peter Norving](https://www.google.com/url?sa=t&rct=j&q=&esrc=s&source=web&cd=1&cad=rja&uact=8&ved=2ahUKEwjYp9Ppru_nAhUBzIUKHfbUAG8QFjAAegQIBhAB&url=https%3A%2F%2Fnorvig.com%2Fbig.txt&usg=AOvVaw2ed9iwhcP1RKUiEROs15Dz).\n",
|
|
"This file contains around 130.000 lines of raw text that will be processed by the library to generate a working tokenizer.\n"
|
|
]
|
|
},
|
|
{
|
|
"cell_type": "code",
|
|
"execution_count": null,
|
|
"metadata": {
|
|
"pycharm": {
|
|
"is_executing": false,
|
|
"name": "#%% code\n"
|
|
}
|
|
},
|
|
"outputs": [],
|
|
"source": [
|
|
"!pip install tokenizers"
|
|
]
|
|
},
|
|
{
|
|
"cell_type": "code",
|
|
"execution_count": 2,
|
|
"metadata": {
|
|
"pycharm": {
|
|
"is_executing": false,
|
|
"name": "#%% code\n"
|
|
}
|
|
},
|
|
"outputs": [],
|
|
"source": [
|
|
"BIG_FILE_URL = 'https://raw.githubusercontent.com/dscape/spell/master/test/resources/big.txt'\n",
|
|
"\n",
|
|
"# Let's download the file and save it somewhere\n",
|
|
"from requests import get\n",
|
|
"with open('big.txt', 'wb') as big_f:\n",
|
|
" response = get(BIG_FILE_URL, )\n",
|
|
" \n",
|
|
" if response.status_code == 200:\n",
|
|
" big_f.write(response.content)\n",
|
|
" else:\n",
|
|
" print(\"Unable to get the file: {}\".format(response.reason))\n"
|
|
]
|
|
},
|
|
{
|
|
"cell_type": "markdown",
|
|
"metadata": {
|
|
"pycharm": {
|
|
"is_executing": false,
|
|
"name": "#%% md\n"
|
|
}
|
|
},
|
|
"source": [
|
|
" \n",
|
|
"Now that we have our training data we need to create the overall pipeline for the tokenizer\n",
|
|
" "
|
|
]
|
|
},
|
|
{
|
|
"cell_type": "code",
|
|
"execution_count": 10,
|
|
"metadata": {
|
|
"pycharm": {
|
|
"is_executing": false,
|
|
"name": "#%% code\n"
|
|
}
|
|
},
|
|
"outputs": [],
|
|
"source": [
|
|
"# For the user's convenience `tokenizers` provides some very high-level classes encapsulating\n",
|
|
"# the overall pipeline for various well-known tokenization algorithm. \n",
|
|
"# Everything described below can be replaced by the ByteLevelBPETokenizer class. \n",
|
|
"\n",
|
|
"from tokenizers import Tokenizer\n",
|
|
"from tokenizers.decoders import ByteLevel as ByteLevelDecoder\n",
|
|
"from tokenizers.models import BPE\n",
|
|
"from tokenizers.normalizers import Lowercase, NFKC, Sequence\n",
|
|
"from tokenizers.pre_tokenizers import ByteLevel\n",
|
|
"\n",
|
|
"# First we create an empty Byte-Pair Encoding model (i.e. not trained model)\n",
|
|
"tokenizer = Tokenizer(BPE())\n",
|
|
"\n",
|
|
"# Then we enable lower-casing and unicode-normalization\n",
|
|
"# The Sequence normalizer allows us to combine multiple Normalizer that will be\n",
|
|
"# executed in order.\n",
|
|
"tokenizer.normalizer = Sequence([\n",
|
|
" NFKC(),\n",
|
|
" Lowercase()\n",
|
|
"])\n",
|
|
"\n",
|
|
"# Our tokenizer also needs a pre-tokenizer responsible for converting the input to a ByteLevel representation.\n",
|
|
"tokenizer.pre_tokenizer = ByteLevel()\n",
|
|
"\n",
|
|
"# And finally, let's plug a decoder so we can recover from a tokenized input to the original one\n",
|
|
"tokenizer.decoder = ByteLevelDecoder()"
|
|
]
|
|
},
|
|
{
|
|
"cell_type": "markdown",
|
|
"metadata": {
|
|
"pycharm": {
|
|
"name": "#%% md\n"
|
|
}
|
|
},
|
|
"source": [
|
|
"The overall pipeline is now ready to be trained on the corpus we downloaded earlier in this notebook."
|
|
]
|
|
},
|
|
{
|
|
"cell_type": "code",
|
|
"execution_count": 11,
|
|
"metadata": {
|
|
"pycharm": {
|
|
"is_executing": false,
|
|
"name": "#%% code\n"
|
|
}
|
|
},
|
|
"outputs": [
|
|
{
|
|
"name": "stdout",
|
|
"output_type": "stream",
|
|
"text": [
|
|
"Trained vocab size: 25000\n"
|
|
]
|
|
}
|
|
],
|
|
"source": [
|
|
"from tokenizers.trainers import BpeTrainer\n",
|
|
"\n",
|
|
"# We initialize our trainer, giving him the details about the vocabulary we want to generate\n",
|
|
"trainer = BpeTrainer(vocab_size=25000, show_progress=True, initial_alphabet=ByteLevel.alphabet())\n",
|
|
"tokenizer.train(trainer, [\"big.txt\"])\n",
|
|
"\n",
|
|
"print(\"Trained vocab size: {}\".format(tokenizer.get_vocab_size()))"
|
|
]
|
|
},
|
|
{
|
|
"cell_type": "markdown",
|
|
"metadata": {
|
|
"pycharm": {
|
|
"name": "#%% md\n"
|
|
}
|
|
},
|
|
"source": [
|
|
"Et voilà ! You trained your very first tokenizer from scratch using `tokenizers`. Of course, this \n",
|
|
"covers only the basics, and you may want to have a look at the `add_special_tokens` or `special_tokens` parameters\n",
|
|
"on the `Trainer` class, but the overall process should be very similar.\n",
|
|
"\n",
|
|
"We can save the content of the model to reuse it later."
|
|
]
|
|
},
|
|
{
|
|
"cell_type": "code",
|
|
"execution_count": 12,
|
|
"metadata": {
|
|
"pycharm": {
|
|
"is_executing": false,
|
|
"name": "#%% code\n"
|
|
}
|
|
},
|
|
"outputs": [
|
|
{
|
|
"data": {
|
|
"text/plain": [
|
|
"['./vocab.json', './merges.txt']"
|
|
]
|
|
},
|
|
"execution_count": 12,
|
|
"metadata": {},
|
|
"output_type": "execute_result"
|
|
}
|
|
],
|
|
"source": [
|
|
"# You will see the generated files in the output.\n",
|
|
"tokenizer.model.save('.')"
|
|
]
|
|
},
|
|
{
|
|
"cell_type": "markdown",
|
|
"metadata": {
|
|
"pycharm": {
|
|
"name": "#%% md\n"
|
|
}
|
|
},
|
|
"source": [
|
|
"Now, let load the trained model and start using out newly trained tokenizer"
|
|
]
|
|
},
|
|
{
|
|
"cell_type": "code",
|
|
"execution_count": 13,
|
|
"metadata": {
|
|
"pycharm": {
|
|
"is_executing": false,
|
|
"name": "#%% code\n"
|
|
}
|
|
},
|
|
"outputs": [
|
|
{
|
|
"name": "stdout",
|
|
"output_type": "stream",
|
|
"text": [
|
|
"Encoded string: ['Ġthis', 'Ġis', 'Ġa', 'Ġsimple', 'Ġin', 'put', 'Ġto', 'Ġbe', 'Ġtoken', 'ized']\n",
|
|
"Decoded string: this is a simple input to be tokenized\n"
|
|
]
|
|
}
|
|
],
|
|
"source": [
|
|
"# Let's tokenizer a simple input\n",
|
|
"tokenizer.model = BPE('vocab.json', 'merges.txt')\n",
|
|
"encoding = tokenizer.encode(\"This is a simple input to be tokenized\")\n",
|
|
"\n",
|
|
"print(\"Encoded string: {}\".format(encoding.tokens))\n",
|
|
"\n",
|
|
"decoded = tokenizer.decode(encoding.ids)\n",
|
|
"print(\"Decoded string: {}\".format(decoded))"
|
|
]
|
|
},
|
|
{
|
|
"cell_type": "markdown",
|
|
"metadata": {
|
|
"pycharm": {
|
|
"name": "#%% md\n"
|
|
}
|
|
},
|
|
"source": [
|
|
"The Encoding structure exposes multiple properties which are useful when working with transformers models\n",
|
|
"\n",
|
|
"- normalized_str: The input string after normalization (lower-casing, unicode, stripping, etc.)\n",
|
|
"- original_str: The input string as it was provided\n",
|
|
"- tokens: The generated tokens with their string representation\n",
|
|
"- input_ids: The generated tokens with their integer representation\n",
|
|
"- attention_mask: If your input has been padded by the tokenizer, then this would be a vector of 1 for any non padded token and 0 for padded ones.\n",
|
|
"- special_token_mask: If your input contains special tokens such as [CLS], [SEP], [MASK], [PAD], then this would be a vector with 1 in places where a special token has been added.\n",
|
|
"- type_ids: If your input was made of multiple \"parts\" such as (question, context), then this would be a vector with for each token the segment it belongs to.\n",
|
|
"- overflowing: If your input has been truncated into multiple subparts because of a length limit (for BERT for example the sequence length is limited to 512), this will contain all the remaining overflowing parts."
|
|
]
|
|
}
|
|
],
|
|
"metadata": {
|
|
"kernelspec": {
|
|
"display_name": "Python 3",
|
|
"language": "python",
|
|
"name": "python3"
|
|
},
|
|
"language_info": {
|
|
"codemirror_mode": {
|
|
"name": "ipython",
|
|
"version": 3
|
|
},
|
|
"file_extension": ".py",
|
|
"mimetype": "text/x-python",
|
|
"name": "python",
|
|
"nbconvert_exporter": "python",
|
|
"pygments_lexer": "ipython3",
|
|
"version": "3.7.6"
|
|
},
|
|
"pycharm": {
|
|
"stem_cell": {
|
|
"cell_type": "raw",
|
|
"metadata": {
|
|
"collapsed": false
|
|
},
|
|
"source": []
|
|
}
|
|
}
|
|
},
|
|
"nbformat": 4,
|
|
"nbformat_minor": 1
|
|
}
|